Table Of Content
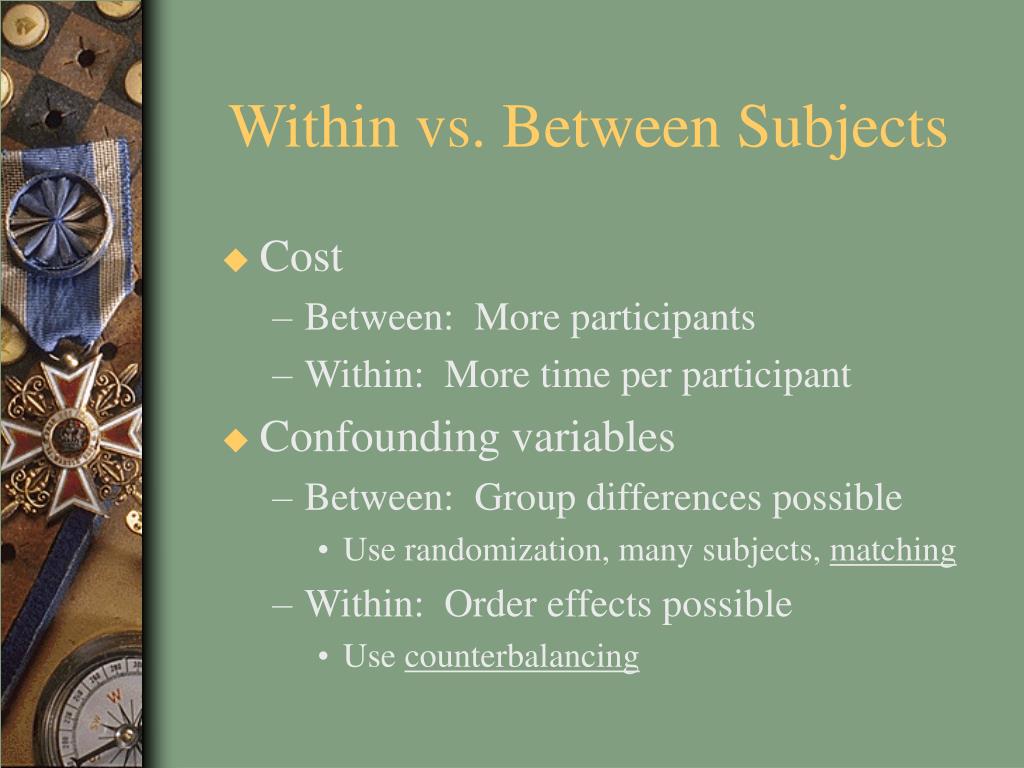
As we will see, interactions are often among the most interesting results in psychological research. Each level of one independent variable is combined with each level of every other independent variable to create different conditions. The left column depicts the predicted proportion of old responses and estimates of recollection and familiarity for Experiment 2a as a function of item type (foil, silent, aloud) or production (silent, aloud). The right column depicts the pairwise contrasts calculated between each of these conditions; thick lines represent the 50% HDI and thin lines represent the 95% HDI. Together, these coefficients can be used to calculate a metric similar to d’ for each group, but again on the logit as opposed to probit scale (we denote the scale of our measure by referring to it as d’L). Following the study phase, participants were tested for their memory of the study items using the remember-know procedure (Tulving, 1985) as described by Ozubko et al. (2012, Experiment 1).
Within-Subjects Design Minimize the Noise in Your Data
How can I analysis a vignette experiment followed a between-subjects design, two-level (unethical consumption vs ... - ResearchGate
How can I analysis a vignette experiment followed a between-subjects design, two-level (unethical consumption vs ....
Posted: Sun, 10 May 2020 07:00:00 GMT [source]
In a between-subjects design, each participant is assigned to only one one level of the independent variable (treatment condition), and researchers will compare group differences between participants in these various conditions. There are no control groups in within-subjects designs because participants are tested before and after independent variable treatments. The pretest is similar to a control condition where no independent variable treatment is given yet, while the posttest takes place after all treatments are administered.
Individual differences may threaten validity
This is because individual differences can contribute more to the variability in the dependent variable, making it harder to detect a significant effect. The pretest posttest design handles several threats to internal validity, such as maturation, testing, and regression, since these threats can be expected to influence both treatment and control groups in a similar (random) manner. For instance, mortality can be a problem if there are differential dropout rates between the two groups, and the pretest measurement may bias the posttest measurement (especially if the pretest introduces unusual topics or content). Each type of experimental design has its own advantages and disadvantages, and it is usually up to the researchers to determine which method will be more beneficial for their study.
Within-Subjects Experiments
For example, a participant who is asked to judge the guilt of an attractive defendant and then is asked to judge the guilt of an unattractive defendant is likely to guess that the hypothesis is that defendant attractiveness affects judgments of guilt. As noted earlier, the production effect is often attributed to a distinctiveness-based strategy at test (“I remember saying it aloud so I must have studied it”). However, participants in our between-subjects experiments commonly reported using this strategy when responding to test items (see the Supplementary Online Materials). Presuming these retrospective reports are accurate, the fact that participants use productive information at test regardless of study design begs the question as to why the production effect is absent for measures of recollection in between-subjects designs. We do not yet have a decisive answer to this challenge, though online strategy judgments would prove useful in determining what precisely participants are doing at test.
A Primer on Foundational Concepts You Need to Start Running Statistical Tests - Towards Data Science
A Primer on Foundational Concepts You Need to Start Running Statistical Tests.
Posted: Sat, 09 Sep 2023 07:00:00 GMT [source]
Researchers can use factorial designs to test multiple independent variables simultaneously. This experimental method combines individualized level of one independent variable with each of other independent variable to come up with varying conditions. In contrast, a mixed factorial design is where one variable is changed between subjects and extra within subjects. In a between-subjects study design, also called independent-groups design, you expose each participant to only one condition of the independent variable. In this type of design, you will typically have a control group and one or more experimental groups.
In this design, participants in one group are exposed to a treatment, a nonequivalent group is not exposed to the treatment, and then the two groups are compared. Imagine, for example, a researcher who wants to evaluate a new method of teaching fractions to third graders. One way would be to conduct a study with a treatment group consisting of one class of third-grade students and a control group consisting of another class of third-grade students. This design would be a nonequivalent groups design because the students are not randomly assigned to classes by the researcher, which means there could be important differences between them.
It becomes rather unlikely that some outside event would perfectly coincide with the introduction of the treatment in the first group and with the delayed introduction of the treatment in the second group. For instance, if a change in the weather occurred when we first introduced the treatment to the patients, and this explained their reductions in depression the second time that depression was measured, then we would see depression levels decrease in both the groups. Similarly, the switching replication helps to control for maturation and instrumentation. Both groups would be expected to show the same rates of spontaneous remission of depression and if the instrument for assessing depression happened to change at some point in the study the change would be consistent across both of the groups. Of course, demand characteristics, placebo effects, and experimenter expectancy effects can still be problems.
With four conditions, there would be 24 different orders; with five conditions there would be 120 possible orders. With counterbalancing, participants are assigned to orders randomly, using the techniques we have already discussed. Thus, random assignment plays an important role in within-subjects designs just as in between-subjects designs. Here, instead of randomly assigning to conditions, they are randomly assigned to different orders of conditions.
Let’s Drive Results Together!
Researchers then analyze these patients and collect data to test their anxiety levels. The psychiatrist can use this study to decide which medication is best for her patients with OCD. They will measure whether the groups differ significantly from each other due to the different levels of the treatment variable that they experienced.
The attractive condition is always the first condition and the unattractive condition the second. Thus any difference between the conditions in terms of the dependent variable could be caused by the order of the conditions and not the independent variable itself. Random assignment is not guaranteed to control all extraneous variables across conditions. The process is random, so it is always possible that just by chance, the participants in one condition might turn out to be substantially older, less tired, more motivated, or less depressed on average than the participants in another condition. One is that random assignment works better than one might expect, especially for large samples.
With between-subject design, this transfer of knowledge is not an issue — participants are never exposed to several levels of the same independent variable. In a between-subjects design (or between-groups, independent measures), the study participants are divided into groups, and each group is exposed to one treatment or condition. In a within-subject design, each participant experiences all experimental conditions, whereas, in a between-subject design, different participants are assigned to each condition, with each experiencing only one condition.
Perhaps the most important advantage of within-subject designs is that they make it less likely that a real difference that exists between your conditions will stay undetected or be covered by random noise. Between-subjects studies require at least twice as many participants as a within-subject design, which also means twice the cost and resources. The differences between the two groups are then compared to a control group that does not receive any treatment. The groups that undergo a treatment or condition are typically called the experimental groups.
And each of these methods has its advantages and disadvantages – one will help to determine the difference between the conditions, but it will take a lot of time, the other will be able to show the differences in different approaches of the subjects. An experimental approach to testing also includes a between groups research design. This approach is based on the fact that two or more groups participate in testing. In the case of any user interface, if we can change the color theme (green or blue) and interactive features like an order form or something else.
No comments:
Post a Comment